This article explores the role of AI in detecting crime trends, how it improves loss prevention efforts, and practical steps for implementation.
What Is AI-Driven Crime Pattern Analysis?
AI-driven crime pattern analysis uses artificial intelligence and machine learning to process large volumes of data from various sources. These sources include incident reports, surveillance records, and even external databases. The system analyzes the data collectively, searching for recurring patterns that might indicate potential threats. Rather than simply flagging isolated incidents, this approach identifies trends over time, enabling organizations to focus their attention on areas where risks are most likely to occur.
How AI Improves Loss Prevention
Real-Time Insights and Early Detection
One of the key advantages of AI-driven crime pattern analysis is its ability to provide real-time insights. When an incident occurs, the system quickly processes the new data and compares it with historical records. This rapid analysis helps identify whether an incident fits a known pattern or represents a new trend that could escalate if not addressed promptly. For example, if multiple thefts occur in a specific area over a short period, the system can alert security teams so that additional measures can be taken immediately.
Early detection through AI means that potential risks are addressed before they develop into larger problems. Quick identification allows organizations to deploy additional resources, preserve evidence, and coordinate responses more effectively. In busy retail or high-traffic environments, this can make a significant difference in reducing losses.
Data-Driven Decision Making
AI systems are capable of processing and analyzing data far more quickly than traditional methods. This capability allows loss prevention teams to base their decisions on current, accurate information rather than outdated reports. The insights generated by the system help in allocating resources where they are most needed, optimizing patrol schedules, or even adjusting store layouts to minimize vulnerabilities.
Data-driven decision making also supports a proactive approach. Instead of waiting for issues to arise, security teams can use AI insights to identify emerging patterns and adjust their strategies accordingly. This shift from reactive to proactive measures can improve overall operational efficiency and reduce the impact of criminal activity.
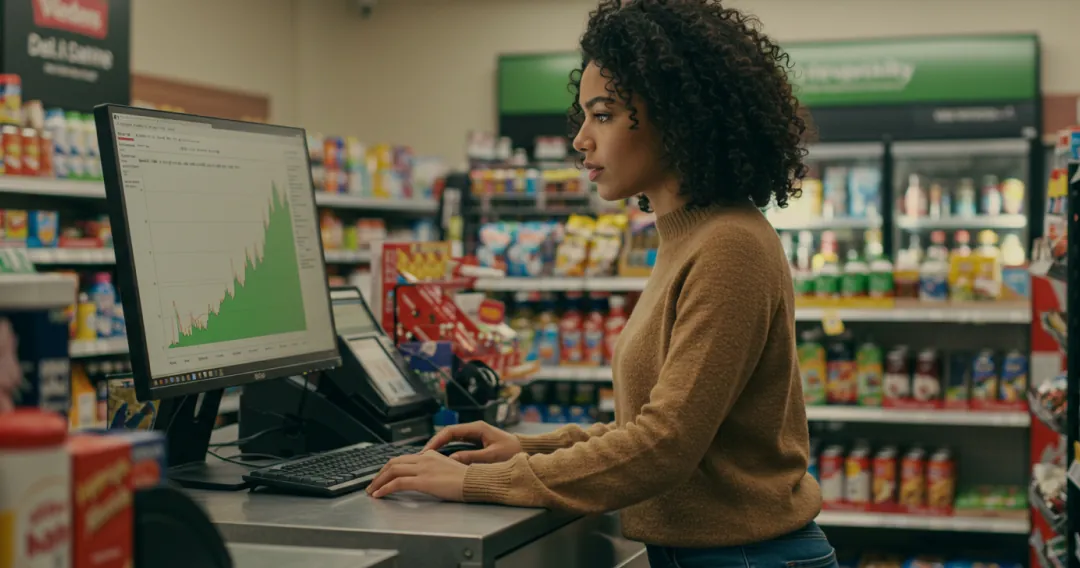
Implementing AI-Driven Crime Pattern Analysis
First Step: Integrate Multiple Data Sources
A critical step in implementing AI-driven analysis is the integration of various data sources. Organizations need to consolidate information from incident reports, surveillance footage, and other relevant records into a single system. This centralization not only simplifies data management but also improves the accuracy of the analysis. When all relevant information is available in one place, the AI algorithms can work more effectively to identify patterns and trends.
Second Step: Set Up Clear Processes
For AI-driven crime pattern analysis to be effective, clear processes must be established. Staff should be trained on how to input accurate data and use the system to monitor incidents. Regular reviews of the system’s performance can help ensure that it continues to provide useful insights. Establishing a routine for data verification and system updates will keep the analysis current and reliable.
Challenges and Considerations
While AI-driven crime pattern analysis offers significant advantages, there are challenges that organizations must address. Data quality is paramount; inaccurate or incomplete data can lead to misleading conclusions. It is essential to ensure that data inputs are reliable and that the system is regularly updated.
Another consideration is the integration of AI systems with existing loss prevention processes. Organizations may need to invest in training and infrastructure to fully leverage the benefits of AI. Additionally, privacy concerns must be managed carefully to ensure that data is handled in accordance with regulatory standards.
Finally, while AI provides valuable insights, human oversight remains crucial. Security teams must interpret the data and decide on appropriate actions. The combination of AI-driven analysis and experienced personnel can lead to more effective loss prevention outcomes.
Final Thoughts
AI-driven crime pattern analysis is transforming how organizations approach loss prevention. By integrating data from various sources and providing real-time insights, this method enables more informed and proactive responses to criminal activity. Although challenges such as data quality and system integration must be addressed, the benefits of faster response times and more accurate risk assessments are clear.
For organizations seeking to improve their security operations, embracing AI-driven analysis is a practical step towards reducing losses and enhancing overall asset protection. With platforms like ThinkLP supporting these efforts, companies can build more resilient systems that adapt to evolving threats and help maintain a secure environment for both assets and customers.
Explore ThinkLP’s Blog
Now that you know more about AI-driven crime pattern analysis, you can find additional insights on loss prevention and safety intelligence on ThinkLP’s blog. The blog features articles, case studies, and industry insights that provide practical tips and strategies for improving your loss prevention efforts.
Request a Demo
If you are interested in how ThinkLP’s software can support your loss prevention initiatives, we invite you to request a demo. Their Loss & Safety Intelligence Platform is designed to integrate with your existing operations, helping you reduce risks and improve efficiency. Reach out today to learn how ThinkLP can assist your organization in optimizing its loss prevention strategy.